Phd #2 at Mines Paris in Data Science - Sophia Antipolis, France - Mines Paris - PSL, Centre PERSEE
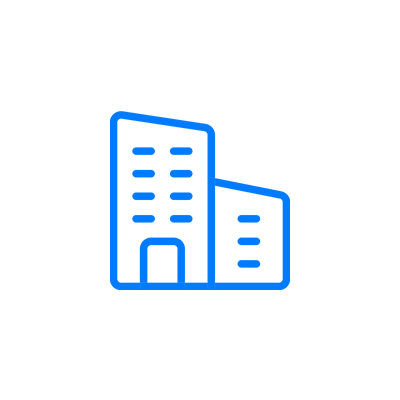
il y a 1 semaine
Description
PhD #2 at Mines Paris in Data Science & Energy: "High-dimensional optimization of distributed assets in smart grids":
- Réf
ABG-111183
- Sujet de Thèse 15/02/2023
- Autre financement public
- Mines Paris
- PSL, Centre PERSEE
- Lieu de travail
- Sophia-Antipolis
- Provence-AlpesCôte d'Azur
- France
- Intitulé du sujet
- PhD #2 at Mines Paris in Data Science & Energy: "Highdimensional optimization of distributed assets in smart grids"
- Champs scientifiques
- Sciences de l'ingénieur
- Energie
- Mathématiques
- Mots clés
- Energy digitalisation, data science, distributed intelligence, optimization, edge analytics, AI on the edge, smart grids, energy transition
Description du sujet:
Title:
"High-dimensional optimization of distributed assets in smart grids"**
Context and background:In the vertically integrated energy systems of the past, power system management was carried out centrally by the transmission and distribution grid operators (TSOs, DSOs). In the frame of energy transition, the paradigm changes towards a decentralized one. This is due to the high number of emerging new actors with different interests (aggregators, microgrid operators, energy community managers, self-consumption etc.), and due to the very high population of assets connected to the grid like renewable energy (RES) plants, storage devices, electric vehicles (EVs), smart-homes/prosumers with IoT devices, electric heating/cooling systems etc. As a function of the involved actors and the related business models, physical or virtual groupings of assets ("cells") may emerge, i.e. virtual power plants (VPP), energy communities, microgrids, a.o. In the power systems of the future most likely all these "cell" variants will coexist. The operation of each such cell is optimized based on the specific interests of the involved actors. For example, a VPP operator aggregates hundreds to thousands of assets to achieve a critical mass of flexibility and valorize it on electricity markets. Optimization functions ("distributed intelligence") are necessary at these lower levels down to the grid edge (cell, feeders, assets/devices ). These functions cannot though be performed independently from the grid. The grid operators may have to send signals for grid-aware control downwards to influence the local optimization processes.
Scientific objectives:
Methodology and expected results:
The first step of the research project is a bibliographic research and familiarization with tools developed at our group.
The initial use case of focus will be the predictive management of the assets (the latter for time frames in the order of a few minutes to a few days ahead).
The developed approaches should be scalable to a very high number of assets to cover use cases like distribution grids or/and Virtual Power Plants (VPPs) that integrate EVs, RES plants, storage devices, prosumers etc.
Each type of asset may have a high inherent uncertainty in its production or consumption profile. Approaches will integrate predictive models that reduce the complexity associated to multiple uncertainties, implementing statistical or machine-learning methods for high-dimension problems (e.g. sparse models, functional data analysis, edge ML).Given that very large amounts of input data are considered, strategies to reduce the computational cost via distributed optimization will be examined.
These strategies will investigate decomposition approaches and optimal decision trees able to adapt dynamically to the incoming information. The high-level signals will be global or locational to account for the local grid conditions (i.e. prediction of congestions/overloads).It will be evaluated how these signals are used in the different cells of assets as a function of the cell optimization objectives.
Prise de fonction:
- 01/05/2023
Nature du financement: - Autre financement public
Précisions sur le financement:
- Project PEPR TASE "AI.
Présentation établissement et labo d'accueil:
- Mines Paris
- PSL, Centre PERSEE
PERSEE Center is one of the 18 research centers of MINES Paris. Its field of expertise concerns New Energy Technologies and Renewable Energy Sources (RES). Its research strategy is based on a "micro/macro" approach ranging from (nano)materials to energy systems.
It is built around three structuring themes:
i) materials and components for energy, ii) sustainable energy conversion and storage processes and technologies, and iii) renewable energies and smart energy systems.
The PERSEE Center is located within the scientific parc of Sophia-Antipolis, near the cities of Nice, Cannes and Antibes in the south of France.
Site web:
Intitulé du doctorat:
- Doctorat en Énergétique et Procédés
Pays d'obtention du doctorat: - France**Etablissement dél
Plus d'emplois de Mines Paris - PSL, Centre PERSEE
-
Optimization of Multi-actor Energy Systems Enhanced
Sophia Antipolis, France - il y a 1 semaine
-
Phd #1 at Mines Paris in Data Science
Sophia Antipolis, France - il y a 1 semaine
-
Phd #2 at Mines Paris in Data Science
Sophia Antipolis, France - il y a 3 jours
-
Intégration de La Ressource Co2 Dans La
Paris, France - il y a 3 jours
-
Phd #1 at Mines Paris in Data Science
Sophia Antipolis, France - il y a 3 jours