Deep Reinforcement Learning for Enhanced Traffic - Champs-sur-Marne, France - Université Gustave Eiffel / IFSTTAR - Site de Marne-la-Vallée
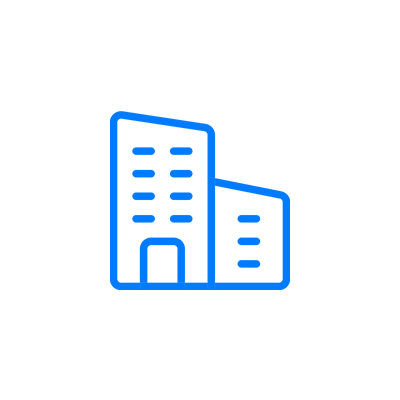
Université Gustave Eiffel / IFSTTAR - Site de Marne-la-Vallée
Champs-sur-Marne, France
Entreprise vérifiée
il y a 2 semaines
Description
Deep Reinforcement Learning for Enhanced Traffic Signal Control with Integrated Drone Detection:
- Réf
ABG-123719 - Sujet de Thèse 07/05/2024
- Contrat doctoral
- Université Gustave Eiffel / IFSTTAR
- Site de MarnelaVallée
- Lieu de travail champs sur marne
- IledeFrance
- France
- Intitulé du sujet
- Deep Reinforcement Learning for Enhanced Traffic Signal Control with Integrated Drone Detection
- Champs scientifiques
- Informatique
- Mathématiques
- Science de la donnée (stockage, sécurité, mesure, analyse)
- Mots clés
- Machine learning, AI, Operation research, Datadriven, computer science, Transport, informatique, mathématiques appliquées,
Description du sujet:
Main host Laboratory:
COSYS-GRETTIA
Main location:
Paris area, France
Doctoral affiliation:
UNIVERSITE GUSTAVE EIFFEL
PhD school:
MATHEMATIQUES ET SCIENCES ET TECHNOLOGIES DE L'INFORMATION ET DE LA COMMUNICATION (MSTIC)
Background
Objectives
- Develop a DRL algorithm for intelligent traffic signal control, integrating drone detection to improve accuracy.
- Investigate the influence of droneassisted detection on DRL's efficiency in traffic signal control.
- Create more realistic and comprehensive traffic management scenarios utilizing drone data.
Methodology
To accomplish the research objectives, the proposed plan entails the following primary steps: (i) A thorough examination of existing DRL techniques, TSC systems, and drone technology in traffic management; (ii) Designing a DRL-based algorithm, incorporating real-time data from drones, and implementing it using Python and relevant libraries; (iii) Evaluate and validate the newly developed model in terms of accuracy, computational efficiency, and applicability in various traffic scenarios.
- The research will be conducted in the following steps:
- Perform a literature review on existing traffic signal control model, RL techniques, and optimization methods in traffic dynamics modeling.
- Develop a Deep RL model for synthetic networks and extend it to realistic scenarios using drone data sets.
- Investigate multiagent communication methods for RLbased algorithms for online traffic control.
- Analyze the impact of the network (intersections) topology on the performance of RL controllers.
- Develop a multiagent RL framework for traffic signal control for multimodal networks, including the incorporation of agents and data collectors (in this case, drones) in the machine learning process.
- Identify and implement the necessary preprocessing steps for extending the framework for multimodal networks.
- Evaluate the performance of the proposed model in various case studies, comparing its accuracy and computational efficiency with existing classic methods, such as Longest queue first and Max pressure, and RLbased approaches for scalable signal control.
References
[1] Wang, T., Cao, J. and Hussain, A., 2021. Adaptive Traffic Signal Control for large-scale scenario with Cooperative Group-based Multi-agent reinforcement learning.
Transportation research part C:
emerging technologies, 125, p
[2] Geroliminis, N., Haddad, J.
, & Ramezani, M Optimal perimeter control for two urban regions with macroscopic fundamental diagrams:
A model predictive approach. IEEE Transactions on Intelligent Transportation Systems, 14(1),
[3] Korecki, M. and Helbing, D., 2021. Analytically guided machine learning for green IT and fluent traffic.
Preprint submitted to
Transportation Research Part C:
Emerging Technologies on, 18.
[4] Abul-Magd, A.Y., 2007. Modeling highway-traffic headway distributions using superstatistics. Physical Review E, 76(5), p
[5] Li, Z., Yu, H., Zhang, G., Dong, S. and Xu, C.Z., 2021. Network-wide traffic signal control optimization using a multi-agent deep reinforcement learning.
Transportation Research Part C:
Emerging Technologies, 125, p
[6] Ducrocq, R. and Farhi, N., 2021. Deep Reinforcement Q-Learning for Intelligent Traffic Signal Control with Partial Detection.
arXiv preprint arXiv:
[7] Alemzadeh S, Moslemi R, Sharma R, Mesbahi M.
Adaptive Traffic Control with Deep Reinforcement Learning:
Towards State-of-the-art and Beyond.
arXiv preprint arXiv:
Jul 21.
[8] Long, M., Zou, X., Zhou, Y. and Chung, E., 2022. Deep reinforcement learning for transit signal priority in a connected environment.
Transportation Research Part C:
Emerging Technologies, 142, p
Prise de fonction:
- 01/09/2024
Nature du financement: - Contrat doctoral
Précisions sur le financement:
Présentation établissement et labo d'accueil:
- Université Gustave Eiffel / IFSTTAR
- Site de MarnelaVallée
It was created on 1 January 2020 as a result of the merger of the University of Paris-Est Marne la vallée, Ifsttar, and several schools and engineering schools:
EIVP, ENSG, ESIEE, and Ecole d'architecture Paris Est. Gusta